/ CASE STUDY
IRIS
How might we improve the speed and accuracy of medical image annotation?
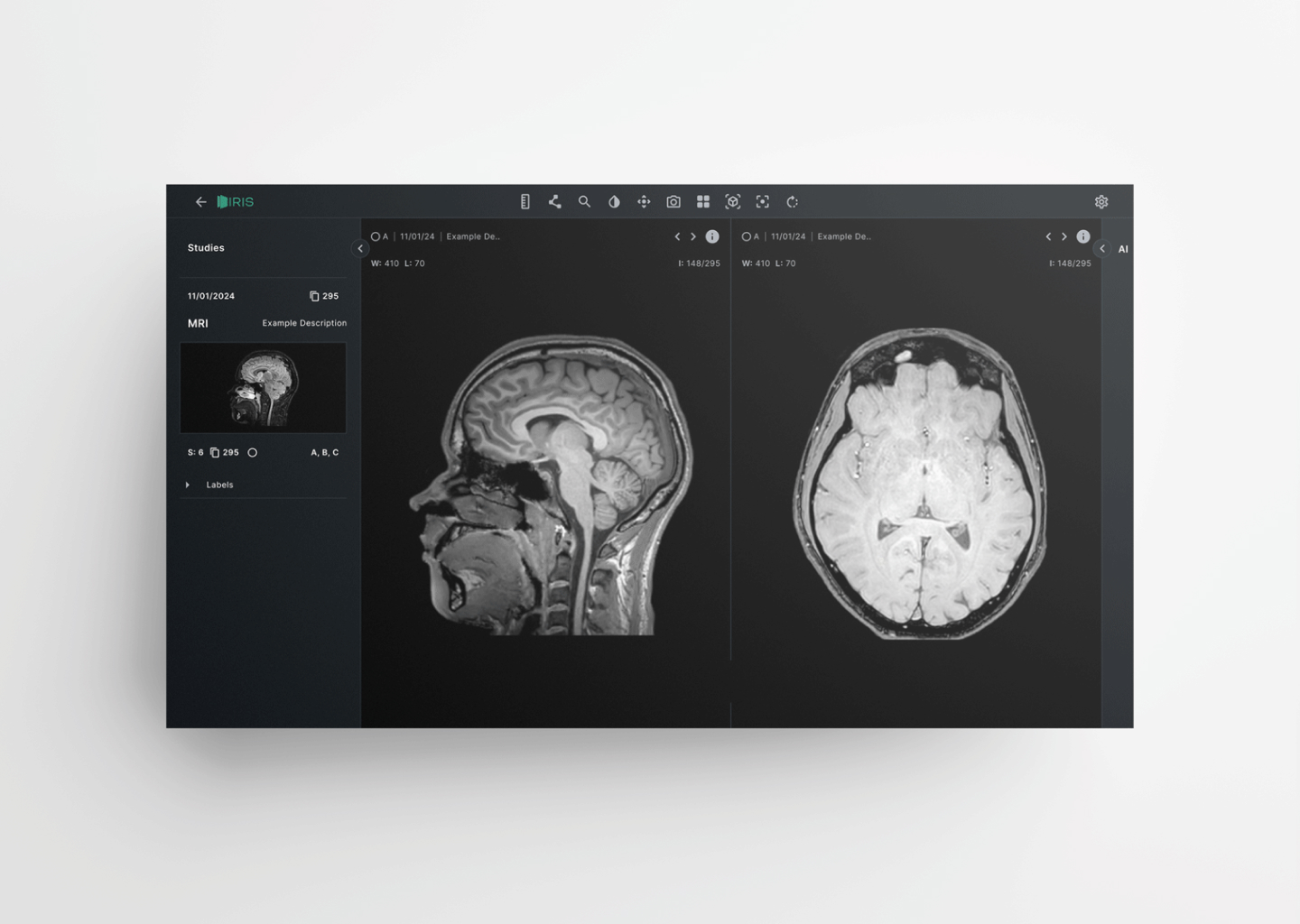
Overview
Medical image annotation is a long and tedious task. Medical Annotators often look through thousands of slice images of any given subject, requiring a well trained eye to find, mark and label any abnormalities. This can potentially mean hours, if not days of work.
However the evolution of AI enables training on extensive datasets of images, teaching itself to autonomously detect abnormalities. I was tasked with designing a new imaging tool for the market that integrates advanced AI capabilities, significantly enhancing the speed and efficiency of radiology annotators' tasks.
However the evolution of AI enables training on extensive datasets of images, teaching itself to autonomously detect abnormalities. I was tasked with designing a new imaging tool for the market that integrates advanced AI capabilities, significantly enhancing the speed and efficiency of radiology annotators' tasks.
Discovery
As there is steadily becoming a standard for medical imaging and DICOM tools, we decided to start our research by analysing competitors. We downloaded and used a variety of different apps that are used to annotate radiology images, as well as talking to radiology professionals to understand their likes and dislikes of various software.
Taking this approach allowed us to quickly form an idea of the best and worst features included in medical imaging tools. We were able to identify similarities between software in order to design a more informed interface that our users would find familiar. This allowed us to get clear about what foundational features we wanted to include in IRIS, and how we could lay those tools out.
Taking this approach allowed us to quickly form an idea of the best and worst features included in medical imaging tools. We were able to identify similarities between software in order to design a more informed interface that our users would find familiar. This allowed us to get clear about what foundational features we wanted to include in IRIS, and how we could lay those tools out.
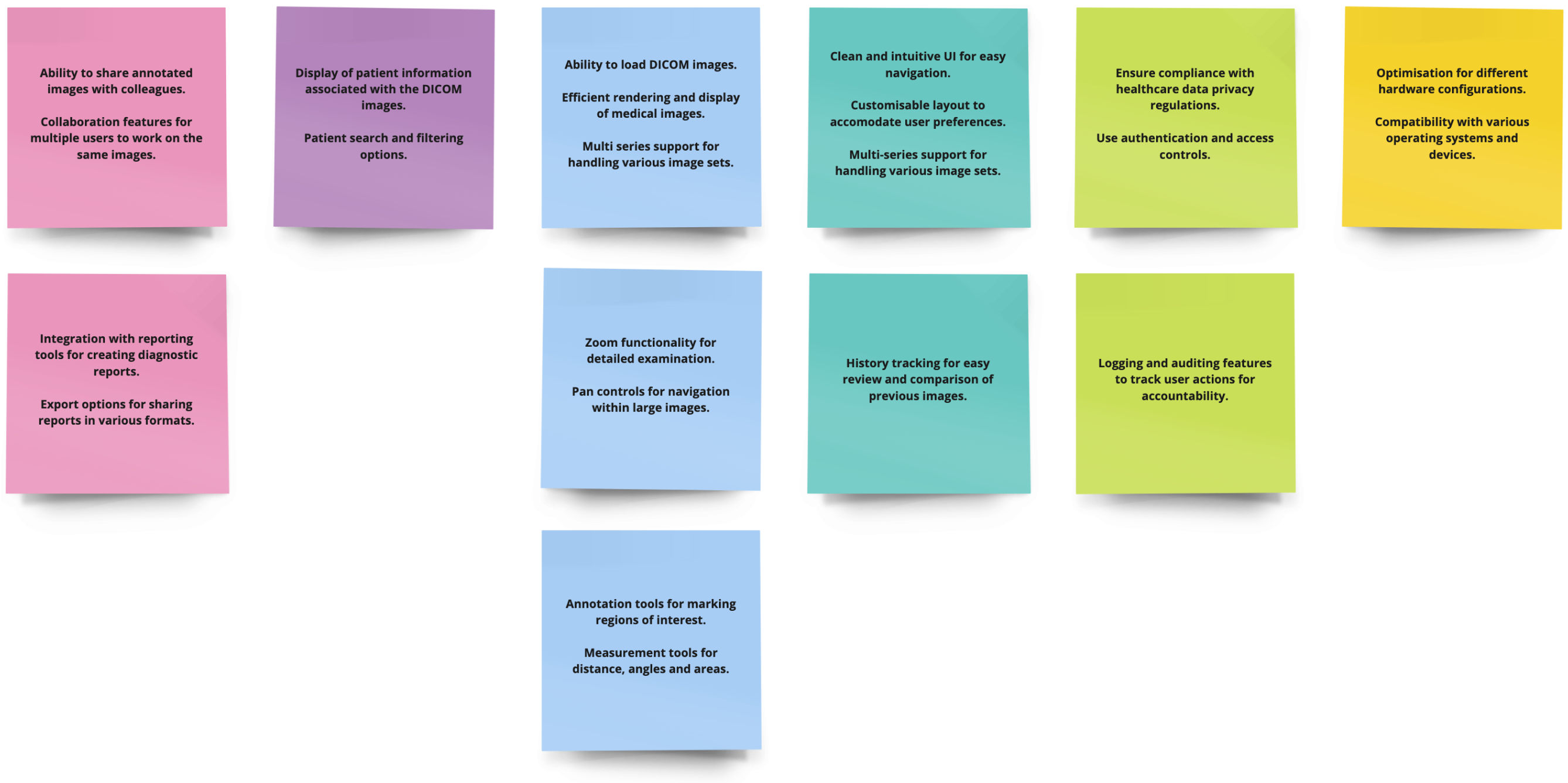
Some of our feature findings from our competitor analysis.
Ideation
With our competitor analysis as a base, we designed wireframe layouts to fit the common standard for DICOM tools, in addition to ideating solutions to incorporate AI overlays into the app. Our AI overlays would work by segmenting parts of the brain using AI technology, so that brain regions and abnormalities can be highlighted with ease, ready for annotation.
We took care to address some usability issues that we found through our research, including:
- Radiology platforms conventionally employ dark colour schemes, an attribute rooted in the necessity of dim working environments used by medical annotators. This could cause some issues with establishing hierarchy within the design and we would need to devise alternative methods of directing attention to crucial areas.
- Additionally, we would need to ensure that the product complied with the principles laid out by the Therapeutic Goods Administration, as TGA approval would be essential for the success of the product.
- Comparison between images is often important for medical annotators, and so we would need some way of using the full screen width to allow up to four images to be shown at one time.
We took care to address some usability issues that we found through our research, including:
- Radiology platforms conventionally employ dark colour schemes, an attribute rooted in the necessity of dim working environments used by medical annotators. This could cause some issues with establishing hierarchy within the design and we would need to devise alternative methods of directing attention to crucial areas.
- Additionally, we would need to ensure that the product complied with the principles laid out by the Therapeutic Goods Administration, as TGA approval would be essential for the success of the product.
- Comparison between images is often important for medical annotators, and so we would need some way of using the full screen width to allow up to four images to be shown at one time.
User Journey Mapping
We broke down the user journey of a medical image annotator and mapped it out in it's simplest form. The User Journey Map made it very easy to visualise
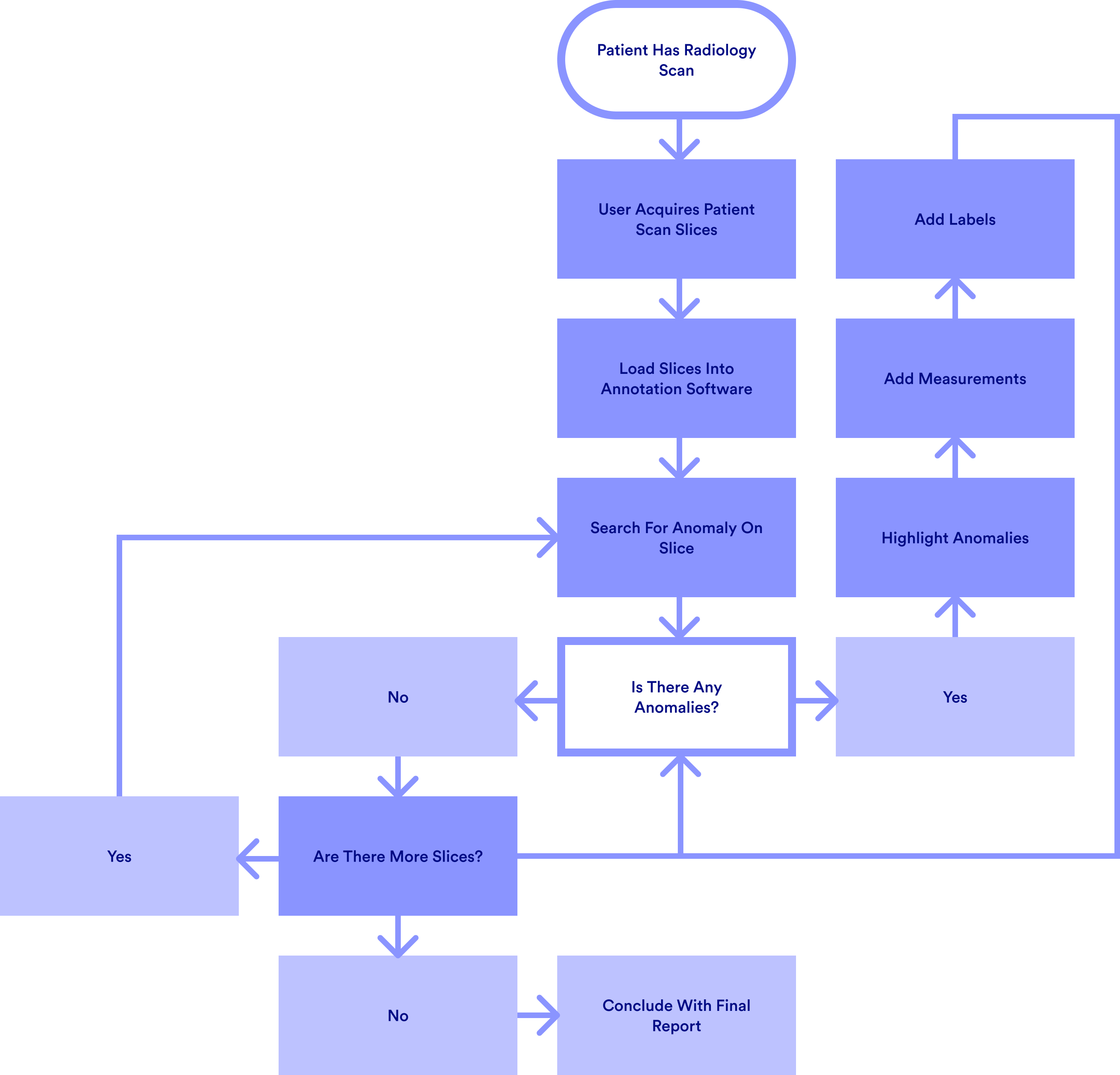
Review
We held a wireframe review with a small group of three radiologists, to discuss the layout and placement of important aspects of IRIS.
During this review we found that overall, the radiologists preferred a layout that allowed the toolbar to sit at the top of the screen, with a slice image sidebar on the left and AI capabilities on the left. We also decided that each sidebar should have the ability to be hidden, allowing for more working screen space.
During this review we found that overall, the radiologists preferred a layout that allowed the toolbar to sit at the top of the screen, with a slice image sidebar on the left and AI capabilities on the left. We also decided that each sidebar should have the ability to be hidden, allowing for more working screen space.
Prototyping & Finalising
We built a working prototype using ProtoPie to simulate loading the slices into IRIS and highlighting a image slice that had been segmented by AI. This allowed us to demonstrate the reduced time required to find and highlight segmented areas of scan slices.
After receiving positive feedback regarding the design and prototype functionality and securing investment from a large network of radiology clinics, we moved towards rolling out an exclusive live beta, with the aim of gathering continuous user feedback to further improve on the design and functionality of the product.
After receiving positive feedback regarding the design and prototype functionality and securing investment from a large network of radiology clinics, we moved towards rolling out an exclusive live beta, with the aim of gathering continuous user feedback to further improve on the design and functionality of the product.
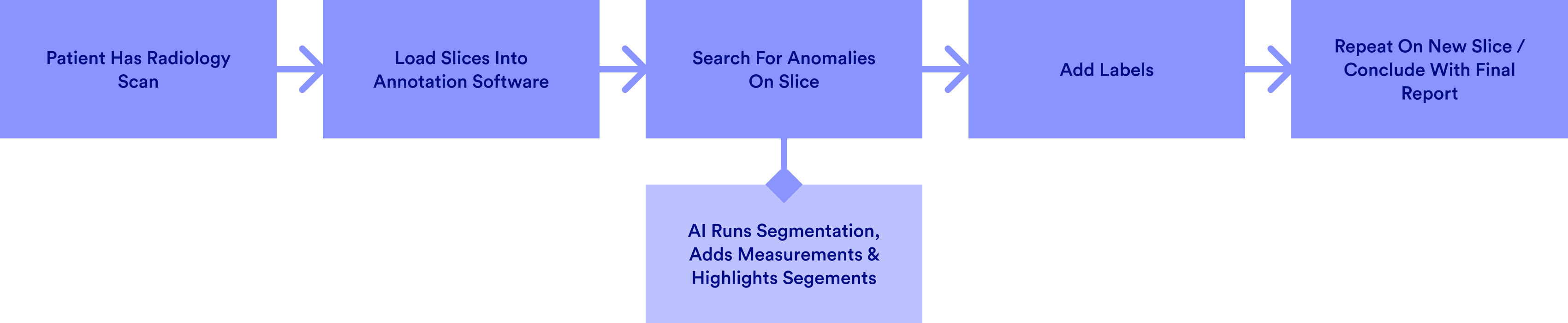
The new user journey when using IRIS.
Using the AI Segmentation feature to highlight the ventricle area of the brain.